Latest from Industry Trends & Research
Closing the Data Divide for a More Equitable US Digital Economy
Technological advances have made it cheaper and easier than ever to collect, process, and use data. This data helps individuals, businesses, and governments make better decisions, and data-driven innovation is a critical pathway for boosting social and economic prosperity. But, in a world in which economic opportunities, government services, and healthcare outcomes are intricately linked to data, how individuals and communities are reflected in datasets and how they can use datasets about themselves significantly impacts their ability to fully participate in the data economy.
The Center of Data Innovation shares a deep dive into data divides in the link at the end of this article. This article is an excerpt from the report sharing how divides are emerging between the data haves and the data have-nots and how these data divides can greatly impact individuals and communities. While many in academia, civil society, and the public sector have considered the impacts of the digital divide, such as disparities in access to broadband, mobile devices, or computers, few have explored the data divide or considered steps to address it.
“…the data divide pertains not only to the quantitative information within datasets themselves, but also to data collection methods. The data divides also relate to system data collection, such as with healthcare and credit data. Data collection can also refer to sensor data collection through IoT-connected devices such as smart appliances or neighborhood security systems.”
What IS the Data Divide?
The data divide refers to the social and economic inequalities that may result from a lack of collection or use of data about individuals or communities. Data divides can manifest in various ways. People in certain places may face greater environmental risks because an insufficient number of sensors gather data about their environmental conditions. Likewise, patients may receive inadequate medical treatments because their demographic is underrepresented in clinical trial data. Other times, some students receive suboptimal educational opportunities because school districts lack the systems to track and measure links between educational programs and outcomes. These data divides can emerge for different reasons, including a lack of resources, political pressure, or legal and regulatory issues.
A simple example is how Americans will be born in hospitals leveraging health informatics, attend schools powered by learning analytics, and live and work in “smart” communities that use data to maximize their economic, social, and environmental prosperity. But others won’t, and the scarcity of data about themselves and their communities will mean that they will not benefit from the advantages of an increasingly data-driven world. These imbalances in data collection and use lead to data divides, and policymakers should prioritize addressing these data inequalities.
The data divide refers to the gap between individuals and communities that have adequate data collected and used about them and those who do not. As the data-driven economy and society continues to develop, those without sufficient data will find that many services work less effectively for them. Consider healthcare: patients without detailed electronic health records (EHRs) will not benefit from health analytics and thus may receive suboptimal care; patients without wearable medical devices will not receive alerts about health abnormalities and thus may not receive life-saving treatments; and patients that are part of groups underrepresented in genetic databases will not benefit from precision medicine. In short, the data divide means that not only will some data-driven services not work for certain people and groups, but data-driven decisions may even be wrong or harmful for them. Without action, a data-driven world will leave some of these individuals and communities behind.
Advances in technology have always created this possibility. Sometimes this is because new technologies are “luxury” items that only upper-income people can afford. Private jets, luxury automobiles, high-end entertainment systems, and other similar products fit into this category. Other times, the technologies are, or at least should be, mass items everyone is able to access, especially as the technologies diffuse through society. Think cell phones, air-conditioning, electricity, and household appliances.
Addressing the data divide requires several considerations of the ideal versus the practical—and not all data divides require equal effort to reduce, nor should they have equal prioritization. In a data-driven world, data equity is what matters, meaning baseline data systems have representative analytics going in and accurate, actionable insights coming out.
Setting Priorities
When data divides impact public goods, closing those gaps should be a top priority. The objective of government is to serve all its constituents. Instances such as government surveys underreaching certain communities or city governments distributing smart sensors unequally should be a high priority. Similarly, the United States should strive for universality for system data, as the ramifications of data divides pose the greatest risk of harm in these areas. For example, a data-driven education system should exist in all communities, regardless of income level. In other areas, such as with wearable smart devices, getting total equality may be costly and ultimately detract from the wider goal.
Electrification initially created a difference in living standards between urban and rural areas. The rise of automobiles created communities with car dependency that made it hard for those without a vehicle to live and work. And the development of the Internet created the digital divide wherein differences in access to information technology (IT), Internet use, and digital skills can create significant disadvantages.
The data divide is similar to these past technological divisions in that the consequence for some is they will have inequitable access to the benefits of the data economy. However, the causes of the data divide, and thus the solutions to it, are unique because data is unlike other goods. Electricity and Internet access are fungible goods. For most consumers, the electricity and Internet service they receive from one provider is identical to that of another. And while someone may greatly prefer a Rolls-Royce over a Kia, in so far as providing basic transportation, vehicles are mostly interchangeable too. But data is not fungible; one set of bits is not the same as another. Giving an individual someone else’s data, such as another person’s health records, does not help them address their own healthcare needs.
“…the data divide means that not only will some data-driven services not work for certain people and groups, but data-driven decisions may even be wrong or harmful for them. Without action, a data-driven world will leave some of these individuals and communities behind.”
These differences matter when it comes to providing solutions. For example, policymakers have sought to close the digital divide by working to increase access and affordability of Internet services and computers. But addressing the data divide will require new thinking about how to collect and make available for use the unique datasets necessary for different individuals and groups to thrive in the data economy.
The data divide is the result of incomplete or missing datasets, including those that are not sufficiently representative of certain populations, cannot be disaggregated to address the needs of different populations, do not address relevant issues, or are not of sufficient quality for a given purpose. Individuals produce a vast amount of data from many different sources, including Internet of Things (IoT)-connected sensors, wearable devices, and payment transactions. Therefore, data divides may have a serious impact on individuals obtaining many of the benefits of using data in sectors such as financial services, environmental monitoring, education, and healthcare. As services increasingly rely on data in the digital economy, disparities will continue to arise between the data haves and the data have-nots.
Moreover, the data divide pertains not only to the quantitative information within datasets themselves, but also to data collection methods. For example, government surveys provide the core collection method for all federal statistics. But data divides also relate to system data collection, such as with healthcare and credit data. Data collection can also refer to sensor data collection through IoT-connected devices such as smart appliances or neighborhood security systems. Addressing the data divide means not only addressing representation issues within statistical surveys but in data collection in all these emerging areas.
In some cases, the social and economic inequalities that may result from this imbalance in data collection and use will be so extreme that some people and groups may experience “data poverty,” wherein a dearth of information on oneself and one’s community has a significantly negative impact on one’s quality of life. Data poverty is somewhat of a phenomenon because what is considered sufficient data changes over time as technologies mature and evolve.
There are two important aspects to the data divide that each matter at both the individual and group levels: data representativeness and data availability. Data quality, whose many dimensions include accuracy, timeliness, precision, and completeness, impacts both data representativeness and data availability. Poor data quality is an important contributing factor to why data divides may persist.
Not Every Data Divide is the Same
Data divides generally fall into one of three categories: system data, geographic data, or demographic data. In each of these categories, data divides may be the result of various causes, including underrepresentation in datasets, invisibility in datasets because the data cannot be disaggregated, poor quality data, and insufficient data for a given purpose.
Underrepresentation in datasets occurs when an individual or community is inadequately reflected in data, be it from counting in federal statistics or geographic placement of data collection technologies. Invisibility in datasets occurs when data is representative but cannot be disaggregated, or separated into sub-categories, thereby obscuring issues only specific groups experience. Poor data quality makes it so that available data cannot be put to practical use. Lastly, a lack of data equity means an individual’s or group’s unique data needs are not being met, as either may require data specific to their circumstances.
System data divides result when there is insufficient data collected in key data systems needed in areas such as education, transportation, healthcare, financial services, and the environment (e.g., credit reporting agencies may not collect data about certain financial activities, making it harder for certain individuals to obtain credit). Geographic data divides result when there is insufficient data about particular places (e.g., rural areas may lack the advanced sensor networks available in urban areas). Demographic data divides result when there is insufficient data about certain populations (e.g., a survey may not be representative of a particular race or age group or obtaining wearable device data may only be affordable for high-income users).
"The data divide refers to the gap between individuals and communities that have adequate data collected and used about them and those who do not. As the data-driven economy and society continues to develop, those without sufficient data will find that many services work less effectively for them."
The Tip of the Data Iceberg
The full report, available at the link below, shares why the data divide in the United States is important to address. It also recommends actions that policymakers should take to ensure the fair and equitable representation and use of data for all Americans.
Below is a summary of the recommendations this report shares about how policymakers can address the data divide:
- Improve federal data quality by developing targeted outreach programs for underrepresented communities. Enhance data quality for non-government data.
- Ensure comparable data collection and monitoring methodologies among the government and civil society.
- Support increased utilization and incorporation of crowdsourced and private-sector data into official datasets.
- Improve documentation and quality of prominent AI datasets to reduce the number of situations with biased results.
- Provide funding from core federal agencies to close both the digital divide and the data divide.
- Direct federal agencies to update or establish data strategies to ensure data collection is integrated into diverse communities.
- Amend the Federal Data Strategy (FDS) to identify data divides and direct agency action.
- Establish a bipartisan federal commission to study the data divide.
Data divides manifest in several ways and should be a top concern for policymakers. The data economy and data-driven innovation present a powerful opportunity to transform society for the better, but only if data collection and use are inclusive. Policymakers should work to ensure that all individuals and communities have access to high-quality data.
REFERENCES AND NOTES
The original, full-length report: https://www2.datainnovation.org/2022-closing-data-divide.pdf
ABOUT THE AUTHOR
Gillian Diebold is a Policy Analyst at the Center for Data Innovation. She holds a B.A. from the University of Pennsylvania, where she studied Communication and Political Science. You can follow her on Twitter @g1lliandiebold.
ABOUT THE COMPANY
The Center for Data Innovation is part of the non-profit, non-partisan Information Technology and Innovation Foundation (ITIF). It conducts independent research and formulates public policies to enable data-driven innovation in the public and private sector. For more information, email [email protected] or visit www.datainnovation.org. Follow them on Twitter @datainnovation, LinkedIn: https://www.linkedin.com/company/center-for-data-innovation/ and Facebook: https://www.facebook.com/CenterForDataInnovation.
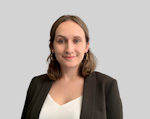
Gillian Diebold | Policy Analyst, Center for Data Innovation
Gillian Diebold is a Policy Analyst at the Center for Data Innovation. She holds a B.A. from the University of Pennsylvania, where she studied Communication and Political Science. You can follow her on Twitter @g1lliandiebold.
The Center for Data Innovation is part of the non-profit, non-partisan Information Technology and Innovation Foundation (ITIF). It conducts independent research and formulates public policies to enable data-driven innovation in the public and private sector. For more information, email [email protected] or visit www.datainnovation.org. Follow them on Twitter @datainnovation, LinkedIn: https://www.linkedin.com/company/center-for-data-innovation/ and Facebook: https://www.facebook.com/CenterForDataInnovation.